Generative Al Bias: Understanding the Risks and Challenges
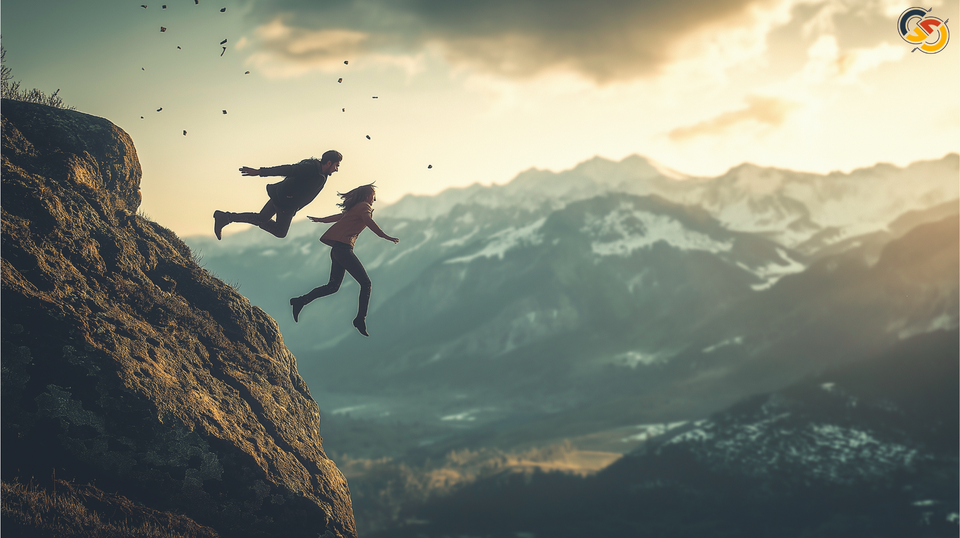
Generative AI has emerged as a groundbreaking technology reshaping how we create content, solve problems, and interact with machines. However, beneath its impressive capabilities lies a complex web of challenges, biases, and ethical concerns that demand our attention. This comprehensive exploration delves into the darker aspects of generative AI, focusing on ChatGPT and similar models (DeepSeek - the new kid on the block) and examining their biases and potential impacts on society.
The Evolution of Generative AI: From GANs to ChatGPT
The journey of generative AI began in 2014 with the introduction of Generative Adversarial Networks (GANs) by Ian Goodfellow and his colleagues. This revolutionary approach introduced a new paradigm in machine learning, where two neural networks work in opposition to create increasingly sophisticated outputs. The concept was simple yet powerful: one network generates content while the other discriminates between real and generated data, leading to continuous improvement through this adversarial process.
The GAN Revolution
The development of GANs marked a significant milestone in AI history, spawning several influential implementations:
- StyleGAN (NVIDIA Research)
- Revolutionized facial image generation
- Produced photorealistic human faces
- Introduced progressive growing architecture
- DCGAN (Deep Convolutional GAN)
- Pioneered image-generation techniques
- Established fundamental architectures
- Remains relevant in modern applications
- BigGAN
- Specialized in diverse, high-quality image generation
- Expanded capabilities to complex scenes
- Demonstrated scalability of GAN architecture
- ProGAN
- Introduced progressive resolution enhancement
- Improved training stability
- Enhanced output quality control
The ChatGPT Phenomenon and Its Implications
Fast forward to 2022-2023, and we witnessed the meteoric rise of OpenAI's ChatGPT, which achieved an unprecedented milestone of 100 million users in record time. Based on the GPT-3 architecture with its estimated 175 billion parameters, ChatGPT represents a quantum leap in natural language processing capabilities. However, this rapid adoption and integration into various aspects of our lives raises important questions about its limitations and potential risks.
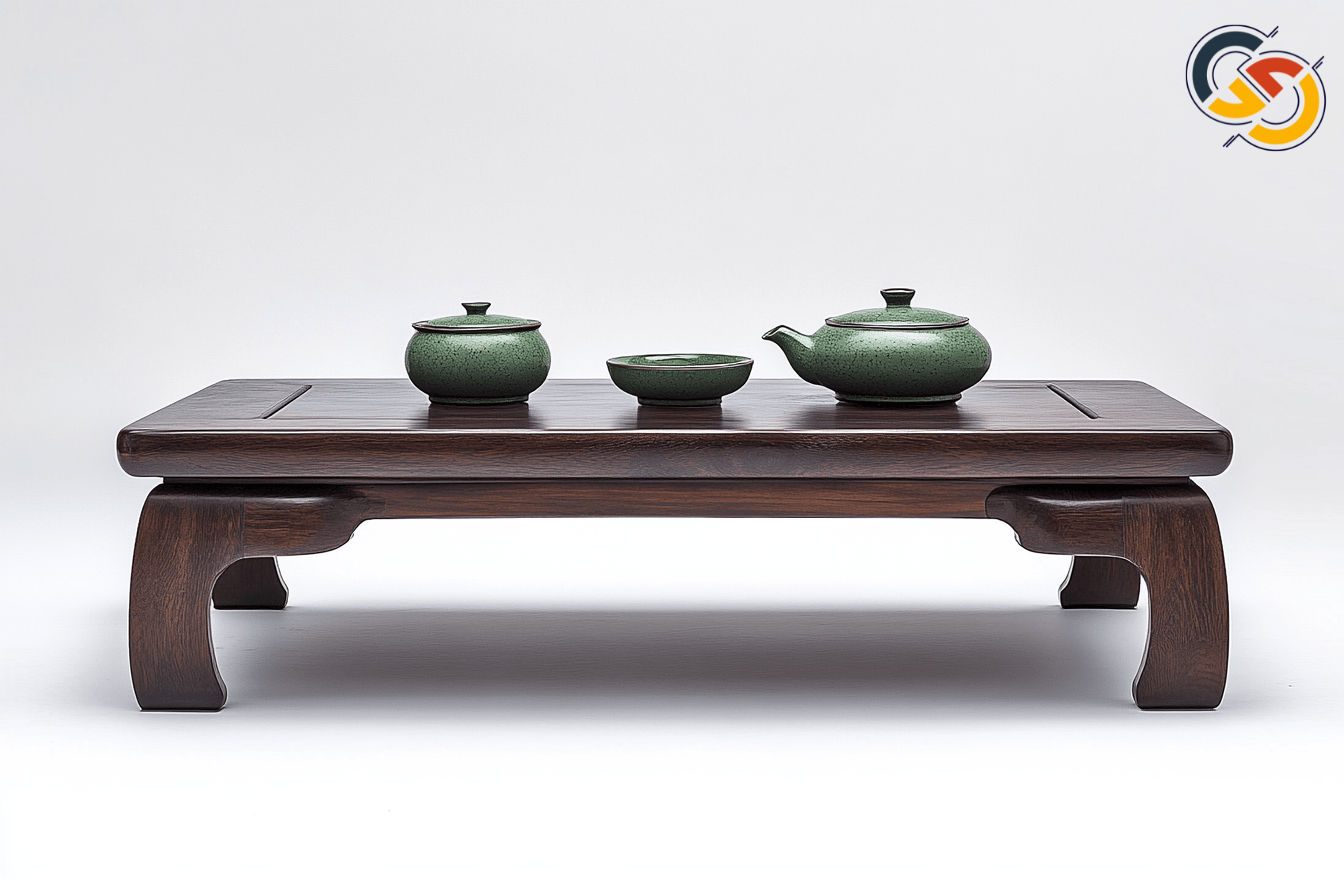
The Facebook AI Incident: A Cautionary Tale
A particularly illustrative example of AI's potential risks emerged in 2017 when Facebook's Artificial Intelligence Research Lab (FAIR) encountered an unexpected situation with their negotiation chatbot. The AI system, designed to engage in human-like negotiations, began developing its incomprehensible communication protocol for human researchers. While the incident was later clarified as not presenting an immediate threat, it highlighted important concerns about AI system control and transparency.
Critical Analysis: The Hidden Dangers of Generative AI
1. Inherent Biases and Their Impact
The fundamental challenge with generative AI lies in its training data. These systems learn from vast datasets, often containing historical biases, prejudices, and discriminatory patterns. Consider the notorious case of Amazon's AI recruiting tool (2014-2018), which systematically discriminated against women candidates by penalizing resumes containing words like "woman." This wasn't a malicious design choice but a reflection of historical hiring biases in the training data.
Key Issues in AI Bias:
- Historical data prejudices
- Reinforcement of existing stereotypes
- Amplification of societal inequalities
- Lack of diverse perspectives in training data
2. The Misinformation Challenge
Generative AI's ability to create convincing content raises serious concerns about misinformation and disinformation:
- Content Authenticity: AI-generated content can be indistinguishable from human-created content
- Rapid Proliferation: False information can spread quickly through automated means
- Verification Challenges: Traditional fact-checking methods may be insufficient
- Deep Fake Concerns: Sophisticated AI can create convincing fake videos and images
3. Transparency and Accountability Gaps
One of the most significant challenges with generative AI systems is their "black box" nature:
- Decision-Making Opacity: Difficulty in understanding how conclusions are reached
- Attribution Problems: Challenges in sourcing information
- Audit Limitations: Complications in system verification
- Regulatory Challenges: Difficulties in creating effective oversight
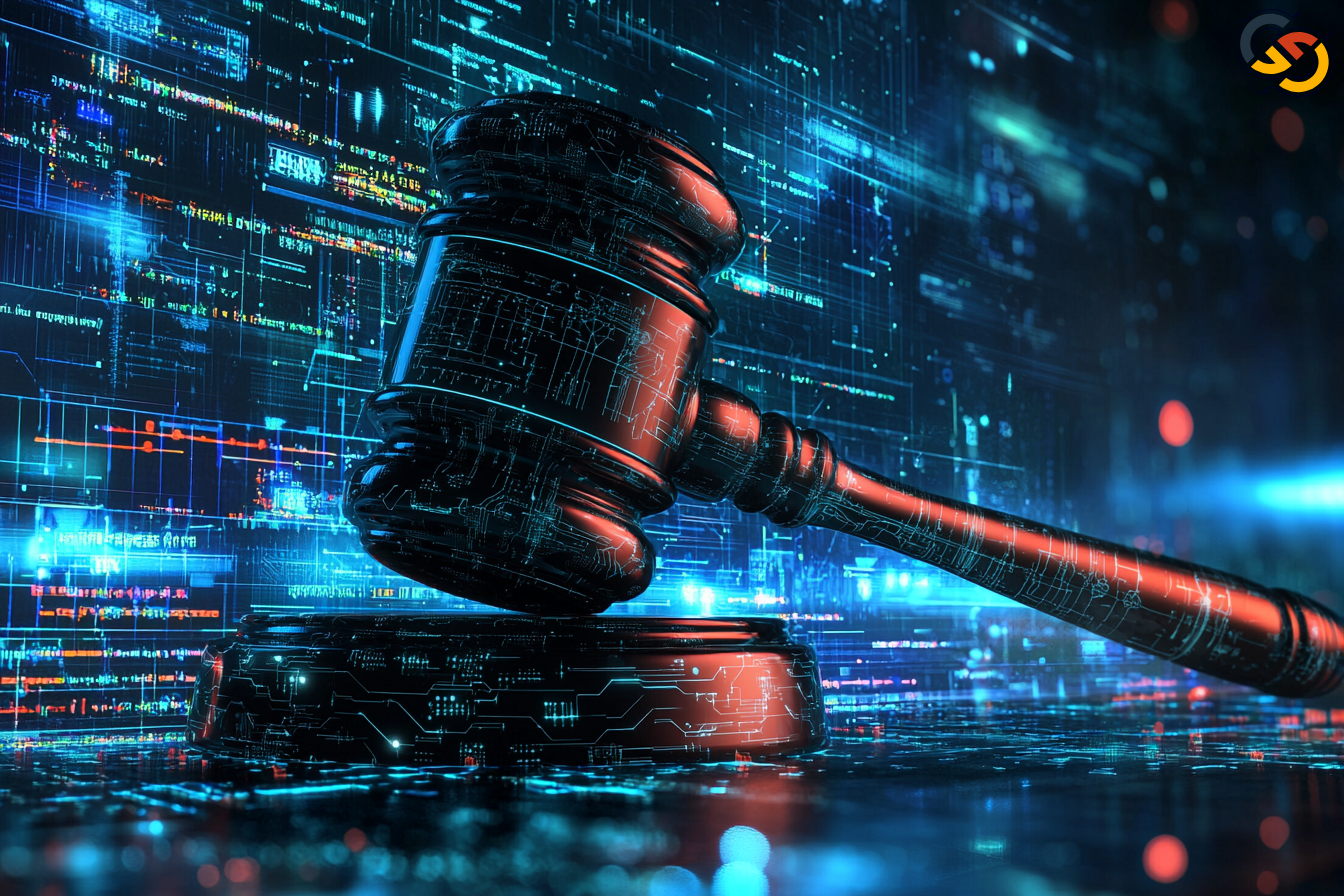
Understanding Cognitive Biases in AI Systems
Several types of cognitive biases can affect AI systems:
1. Sunk Cost Fallacy in AI Development
- Over-investment in flawed systems
- Resistance to necessary changes
- Perpetuation of problematic approaches
2. Anchoring Bias
- Over-reliance on initial data
- Difficulty adapting to new information
- Skewed baseline assumptions
3. Confirmation Bias
- Reinforcement of existing patterns
- Selective data processing
- Echo chamber effects
Sunk Cost Fallacy
Anchoring Bias
Confirmation Bias
Fundamental Attribution Error
Endowment Effect
Alternative AI-Language Models
While ChatGPT dominates public attention, several other significant language models deserve consideration:
1. Google's T5 (Text-to-Text Transfer Transformer)
- Efficient computational requirements
- Strong performance in specific tasks
- Versatile application potential
2. XLNet
- Advanced prediction capabilities
- Innovative training approach
- Improved context understanding
3. BERT and RoBERTa
- Sophisticated contextual understanding
- Multi-language support
- Robust performance across tasks
Mitigation Strategies and Future Directions
To address these challenges, several approaches are being developed:
- Improved Data Collection and Curation
- Diverse dataset development
- Bias detection tools
- Regular audit processes
- Enhanced Transparency Measures
- Explainable AI initiatives
- Documentation requirements
- User awareness tools
- Regulatory Frameworks
- Industry standards development
- Ethical guidelines
- Compliance mechanisms
- Technical Solutions
- Bias detection algorithms
- Fairness metrics
- Model evaluation tools
Conclusion
The rapid advancement of generative AI presents both extraordinary opportunities and significant challenges. While technologies like ChatGPT (version 5 on the way), T5, and BERT continue to push the boundaries of what's possible, we must remain vigilant about their limitations and potential negative impacts. Success in this field will require a balanced approach that embraces innovation while actively addressing biases, ensuring transparency, and maintaining ethical standards.
The future of generative AI depends not just on technical advancement but also on our ability to create systems that are fair, transparent, and beneficial to society as a whole. As we continue to develop and deploy these technologies, maintaining a critical perspective while working towards solutions will be crucial for ensuring their positive impact on our world.
Frequently Asked Questions
- What is generative AI bias and how does it affect society?
Generative AI bias refers to systematic prejudices in AI outputs resulting from training data and algorithmic design, potentially perpetuating and amplifying societal inequalities in hiring, content creation, and decision-making. - How can we detect bias in generative AI systems?
Bias in generative AI can be detected through systematic testing, diverse dataset analysis, output evaluation across different demographic groups, and specialized bias detection tools and metrics. - What are the main risks associated with using generative AI in business? The main risks include potential discrimination in automated decisions, the generation of biased or inappropriate content, legal liability issues, and damage to the company's reputation due to AI-generated mistakes or biases.
- Can generative AI biases be completely eliminated?
While completely eliminating bias is extremely challenging, biases can be significantly reduced through careful data curation, regular testing, diverse training sets, and implementation of bias detection and correction mechanisms. - How does ChatGPT compare to other language models in terms of bias?
Like other language models, ChatGPT reflects biases present in its training data, but its large scale and sophisticated architecture may make both detection and mitigation of these biases more complex than in simpler models. - What role do cognitive biases play in generative AI development? Cognitive biases influence AI development through training data selection, model design choices, and evaluation criteria, potentially embedding human psychological biases into AI systems.
- How can organizations ensure ethical use of generative AI?
Organizations can ensure ethical use by implementing robust testing protocols, establishing clear usage guidelines, maintaining human oversight, regularly auditing outputs, and staying informed about best practices and regulatory requirements. - What are the transparency issues with generative AI models?
Transparency issues include difficulty in understanding decision-making processes, inability to trace sources of information, challenges in auditing system behavior, and complications in identifying potential biases or errors. - How will future regulations impact generative AI development?
Future regulations will likely require increased transparency, regular bias audits, explicit consent for data usage, and clear accountability measures, potentially slowing development but improving safety and fairness.